Speaker Introduction- Dr Neel Madhukar
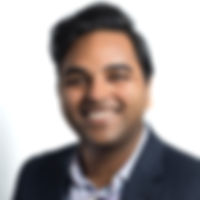
Neel received his PhD from Weill Cornell Graduate School, where his research focused on designing new machine learning approaches for biological problems. At Weill Cornell, he founded OneThree Biotech, with his work culminating in the development of new AI prediction methods, the discovery of a novel class of cancer therapeutics, and the start of new clinical trials.
OneThree Biotech: What is it?
OneThree seeks to find new cures for patients through an AI-discovery drug platform. The platform has been used to identify and validate new mechanisms, candidates and combination therapies for diseases such as breast cancer, pheochromocytoma and resistant gastric cancers.
Vision - to better lives by deciphering the mysteries of disease biology
Mission - to transform how new medicines are discovered using AI to translate the world's data into actionable biology
Beginning in the 2010s at Weill Cornell’s Precision Medicine, Dr Madhukar’s team saw the emergence of computational methods from the world of technology, but little application to biological data. Therefore, they sought to show the world how AI can impact drug discovery and dominate the future of the field.
From 2011-onwards OneThree has worked with different pharma and biotech companies, such as AstraZeneca, Jubilant Therapeutics and Oncoceutics, to deal with drug discovery related problems.
How does OneThree’s approach differ from the traditional approach to drug discovery?
Traditional drug development is thought of as a linear process:
Targets and mechanisms → build a treatment → test on patients
This formula is rigid and strictly enforces researchers to stick to the one linear stage process. Within 30-40 years this process for drug development has been left unchanged, leaving many questions unanswered and pushed to later stages, as well as not isolating specific problems in new drugs which could be altered.
OneThree uses a modular approach:
Dr Madhukar and his team staunchly refute the linear approach, opting for a more ‘wibbly wobbly’ approach to drug discovery. Instead, they use a modular approach, as shown below.

With this approach, every stage of drug development is shown to interlink and throughout the drug development process one must take into consideration all stages.
By focusing on biological questions and their interlinkages, rather stage-dependent problems, developers get a better understanding of the underlying biology of patients and the relationship with the developing drug. From here companies’ ability to develop and generate solutions is made more efficient.
OneThree's Data Engine
OneThree focuses not just on the what but the why.
How do they do this?
Using both data and models, they formulate their unique data engine, which takes data from different sources: the public domain; from pharma partners; and data generated themselves (proprietary data). This data is then formulated and filtered by a data engineering team.
Although massive datatypes each come with their own inherent problems, by focusing not just on the amount of data, but on the ability to link disparate data types and sources together, biological solutions can be found.
Simply looking at problems won’t solve anything, but considering how to build them together and across all data makes this massive data compilation useful: it helps overcome data interpretability and accuracy problems experienced when considering just one data type.

OneThree's AI Ethos
Biology shouldn’t be forced into an AI box: OneThree doesn’t believe in a one-size fits all approach. Instead, using biotech, we must build approaches customised for each problem. Biology is complex and needs to be modelled in this way.
Due to this, OneThree works on designing ensemble models to accommodate input data diversity.
Benefits of ensemble workflow:
By combining all ensemble models together, higher accuracy is achieved, and mechanistic insights better understood (answering ‘why’, not just ‘what’).
More data diversity is enabled, as there are no constraints due to data insights of one particular model
Each dataset is imperfect. There are two ways to go about this:
1) Try and generate perfect datasets. However, this requires a lot of funding and is almost unfeasible to reach
2) Fill in gaps into a mechanistic workflow, as done by OneThree. This requires less funding and uses AI methods more efficiently in achieving a solution.
Case Studies
The best way to demonstrate the effectiveness of OneThree’s ensemble workflow approach is through case studies, which demonstrate how AI methods have been applied in practice and the benefits of using AI in drug development.
CASE STUDY 1: Oncoceutics success story
Problem: MoA Discovery and Positioning for ONC201
Oncoceutics discovered ONC201 through a phenotypic screen for p53 integrated stress response; however, they were unable to discover the anti-cancer mechanism and were stuck at phase II trials.

OneThree used different AI methodologies to figure out 1) why it works 2) how to use that information to pinpoint specific patients for future trials
Mechanistic approach overview
Using OTB Target Deconvolution module OneThree ranked all possible target MoAs for ONC201
Input Data Sets
Chemical structure of ONC201
Efficacy screening against 60 cancer cell lines
Post treatment gene expression changes
2. From there OneThree ran an algorithm which found that the top ranked prediction for ONC201’s MoA was selective antagonism against DRD2 (a novel anti-cancer target)
3. DRD2 binding prediction was validated through preclinical assays and clinical readouts.
Pinpointing H3K27 GBM as an Optimal indication
Using the OTB Gene module, OneThree’s team ranked how susceptible 300 different cancer subtypes would be to DRD2 inhibition
Based on this ranking, they identified glioblastoma and pheochromocytoma as ideal areas for clinical development
Here they could narrow the patient population affected most to those with H3K27 methylation, which was identified as a predictive biomarker for ONC201 treatment in glioblastoma.
The pinpointing of H3K27 was clearly driven by mechanistic approach. This approach enabled OneThree to help Oncoceutics to deconvolute the predictions and give them the details of each step of clinical trial process. This provided an explanation of the problem and why it was present.
From here they were able to validate this prediction and work with Oncoceutics to start new trials in Ph II trial for H3K27M GBM.
Results from the Ph II Trial for H3K27 GBM
Prior to this the patient population had a life expectancy from 3-6 months. After OneThree and Oncoceutics' new trial, the patient population were found to be stable and disease free for over 2 years
The trial also saw the shrinking of tumours in their patient population
The process was a milestone, being one of the first AI based drug discovery validated, as well as seeing direct patient impact by mechanistic AI approaches
The Power of AI
“OneThree’s platform helped solve problems that we had been working on for years and potentially saved several years of additional work. I expect that this approach will be integral to the future of drug discovery”
Joshua Allen, PhD (Chief Scientific Officer, Oncoceutics)
OneThree’s Biotech methods highlighted the efficiency of using AI in the drug discovery process. Their platform provided insights within 3 months, giving direct impact and results compared to the 4 years of unsuccessful insights Oncoceutics had while using traditional techniques.
However, Dr Madhukar notes that AI isn’t set out to replace traditional drug discovery, but to focus on the questions and insights traditional methods couldn’t answer.
Success after the use of biotech
Oncoceutics’ further success after is highlighted by the fact that they were acquired by Chimerix for approximately $400 million soon after the successful treatment. This exemplifies the huge financial impact of OneThree’s AI methods and how every player reaped benefits.
CASE STUDY 2: Predicting organ specific side effects for small molecules
Problem: Unknown side effects are the cause of over 50% clinical failures and withdrawals.
Solution: Developed an AI method to predict the likelihood of severe side effects and the underlying mechanism.
Here OneThree focused on data diversity and on integrating data on tissue level, as well as looking on a patient level data to build their own biological network.
By integrating more data and combining deep learning methods to form an ensemble, they could predict specific side effects for each tissue. Here, they could clearly see the inaccuracies within each tissue
Results – their team accurately predicted specific side effects for over 80% of all approved and withdrawn drugs. OneThree’s data driven approach captured differences between drugs which wouldn’t have been seen in a traditional discovery approach.
Power of AI
An AI based platform goes further than simply identifying the drugs side effects and explains what is driving the toxicity of that drug. AI provides crucial information for pharma companies in isolating the specific stage in the drug development process which makes the drug toxic and how partner companies can reach that stage and act on this information to minimise the side effects.
Scepticism of deep learning - should we stop using traditional methods?
Although Dr Madhukar provides a critical stance on deep learning, he doesn’t believe there should be a complete termination of the usage of traditional methods, such as deep learning. He posits that there should just be more awareness on the positive impacts AI platforms can bring to drug discovery.
It therefore depends on what using deep learning is being used for. If its function is to understand a chemical structure and is image-based, deep learning is ideal.
However, using drug repositioning and genomic data, why would deep learning be the best approach?
We shouldn't throw out deep learning, but must show that there is no magical fix to each problem.
"Data is what you make of it." - Dr Neel Madhukar
More information can be found here:
@onethreebiotech